18新利亚洲|18新利维护
Skip to content THE SOURCE Close TopicsTopics Arts & Culture Business & Entrepreneurship Campus & Community Humanities & Society Medicine & Health Science & Technology SchoolsSchools Arts & Sciences Brown School McKelvey School of Engineering Olin Business School Sam Fox School of Design & Visual Arts School of Continuing & Professional Studies School of Law School of Medicine PublicationsPublications Newsroom The Record Washington Magazine Search Menu Search for: Search Close NEWSROOM Sections Find an Expert Media Resources Newsroom Stories Perspectives WashU Experts WashU in the News New machine learning method can better predict spine surgery outcomes Researchers who had been using Fitbit data to help predict surgical outcomes have a new method to more accurately gauge how patients may recover from lower back surgery. (Photo: Shutterstock) By Leah Shaffer June 3, 2024 SHARE Researchers who had been using Fitbit data to help predict surgical outcomes have a new method to more accurately gauge how patients may recover from spine surgery. Using machine-learning techniques developed at the AI for Health Institute at Washington University in St. Louis, Chenyang Lu, the Fullgraf Professor at the university’s McKelvey School of Engineering, collaborated with Jacob Greenberg, MD, an assistant professor of neurosurgery at the School of Medicine, to develop a way to more accurately predict recovery from lumbar spine surgery. The results, published this month in the journal Proceedings of the ACM on Interactive, Mobile, Wearable and Ubiquitous Technologies, show that their model outperforms previous models to predict spine surgery outcomes. This is important because in lower back surgery and many other types of orthopedic operations, outcomes vary widely depending on the patient’s structural disease but also on varying physical and mental health characteristics across patients. Surgical recovery is influenced by both physical and mental health before the operation. Some people may have excessive worry in the face of pain that can make pain and recovery worse. Others may suffer from physiological problems that worsen pain. If physicians can get a heads-up on the various pitfalls a patient faces, they can better tailor treatment plans. “By predicting the outcomes before the surgery, we can help establish some expectations and help with early interventions and identify high risk factors,” said Ziqi Xu, a PhD student in Lu’s lab and first author on the paper. Previous work in predicting surgery outcomes typically used patient questionnaires given once or twice in clinics, capturing a static slice of time. “It failed to capture the long-term dynamics of physical and psychological patterns of the patients,” Xu said. Prior work training machine-learning algorithms focused on just one aspect of surgery outcome “but ignored the inherent multidimensional nature of surgery recovery,” she added. Getting a ‘big picture’ view Researchers have used mobile health data from Fitbit devices to monitor and measure recovery and compare activity levels over time. But this research has shown that activity data, plus longitudinal assessment data, is more accurate in predicting how the patient will do after surgery, Greenberg said. The current work offers a “proof of principle” showing that, with multimodal machine learning, doctors can see a more accurate “big picture” of the interrelated factors that affect recovery. Before beginning this work, the team first laid out the statistical methods and protocol to ensure they were feeding the artificial intelligence system the right balanced diet of data. Previously, the team had published work in the journal Neurosurgery showing for the first time that patient-reported and objective wearable measurements improve predictions of early recovery compared to traditional patient assessments. In addition to Greenberg and Xu, Madelynn Frumkin, a PhD student studying psychological and brain sciences in Thomas Rodebaugh’s laboratory in Arts & Sciences, was co-first author on that work. Wilson “Zack” Ray, MD, the Henry G. and Edith R. Schwartz Professor of neurosurgery at the School of Medicine, was co-senior author, along with Rodebaugh and Lu. Rodebaugh is now at the University of North Carolina at Chapel Hill. In that research, they show that Fitbit data can be correlated with multiple surveys that assess a person’s social and emotional state. They collected that data via “ecological momentary assessments” (EMAs) that employ smartphones to give patients frequent prompts to assess mood, pain levels and behavior multiple times throughout day. “We combine wearables, EMA and clinical records to capture a broad range of information about the patients, from physical activities to subjective reports of pain and mental health, and to clinical characteristics,” Lu said. Greenberg added that state-of-the-art statistical tools that Rodebaugh and Frumkin have helped advance, such as “Dynamic Structural Equation Modeling,” were key in analyzing the complex, longitudinal EMA data. Aim to improve long-term outcomes For the most recent study, they took all those factors and developed a new machine-learning technique of “Multi-Modal Multi-Task Learning” to effectively combine these different types of data to predict multiple recovery outcomes. In this approach, the AI learns to weigh the relatedness among the outcomes while capturing their differences from the multimodal data, Lu added. This method takes shared information on interrelated tasks of predicting different outcomes and then leverages the shared information to help the model understand how to make an accurate prediction, according to Xu. It all comes together in the final package, producing a predicted change for each patient’s post-operative pain interference and physical function score. Greenberg said the study is ongoing as the researchers continue to fine-tune their models so they can take more detailed assessments, predict outcomes and, most notably, “understand what types of factors can potentially be modified to improve longer-term outcomes.” Xu Z, Zhang J, Greenberg JK, Frumkin M, Javeed S, Zhang JK, Benedict B, Botterbush K, Rodebaugh TL, Ray WZ, Lu C. 2024. Predicting Multi-dimensional Surgical Outcomes with Multi-modal Mobile Sensing: A Case Study with Patients Undergoing Lumbar Spine Surgery. Proc. ACM Interact. Mob. Wearable Ubiquitous Technol. 8, 2, Article 81 (May 2024) https://doi.org/10.1145/3659628 Greenberg JK, Frumkin M, Xu Z, Zhang J, Javeed S, Zhang JK, Benedict B, Botterbush K, Yakdan S, Molina CA, Pennicooke BH, Hafez D, Ogunlade JI, Pallotta N, Gupta MC, Buchowski JM, Neuman B, Steinmetz M, Ghogawala Z, Kelly MP, Goodin BR, Piccirillo JF, Rodebaugh TL, Lu C, Ray WZ. Preoperative Mobile Health Data Improve Predictions of Recovery From Lumbar Spine Surgery. Neurosurgery. 2024 Mar 29. https://doi.org/10.1227/neu.0000000000002911 This study was funded by grants from AO Spine North America, the Cervical Spine Research Society, the Scoliosis Research Society, the Foundation for Barnes-Jewish Hospital, Washington University/BJC Healthcare Big Ideas Competition, the Fullgraf Foundation, and the National Institute of Mental Health (1F31MH124291-01A). SHARE TopicsBiologyEngineeringMedicineMental HealthScience & Technology Schools McKelvey School of EngineeringRead more stories from McKelvey School of EngineeringVisit McKelvey School of EngineeringSchool of MedicineRead more stories from School of MedicineVisit School of Medicine Leave a Comment Comments and respectful dialogue are encouraged, but content will be moderated. Please, no personal attacks, obscenity or profanity, selling of commercial products, or endorsements of political candidates or positions. We reserve the right to remove any inappropriate comments. We also cannot address individual medical concerns or provide medical advice in this forum. You Might Also Like AI for Health Institute launches to promote growing intersection of artificial intelligence, health October 18, 2023 Published In Newsroom Stories Interdisciplinary team researches potential treatments for intervertebral disc disease July 13, 2021 Published In Newsroom Stories Five factors to ensure an infant thrives March 18, 2024 Published In Newsroom Stories Latest from the Newsroom Recent Stories Reframing voting as ‘duty to others’ key to increasing engagement, turnout Modifying homes for stroke survivors saves lives, extends independence Book explores consequences of political conversations WashU Experts Ten Commandments display probably not legal Social workers key to psychedelic-assisted therapies DeFake tool protects voice recordings from cybercriminals WashU in the News NFL faces ‘Sunday Ticket’ lawsuit: Here’s what’s at stake for the league Transcript: Ezra Klein Interviews Yanna Krupnikov The brain has a waste removal system and scientists are figuring out how it works Publications Washington Magazine Newsroom Record Explore Bookshelf Video Gallery Connect Media Resources Contact Facebook Instagram ©2024 Washington University in St. Louis Go back to top
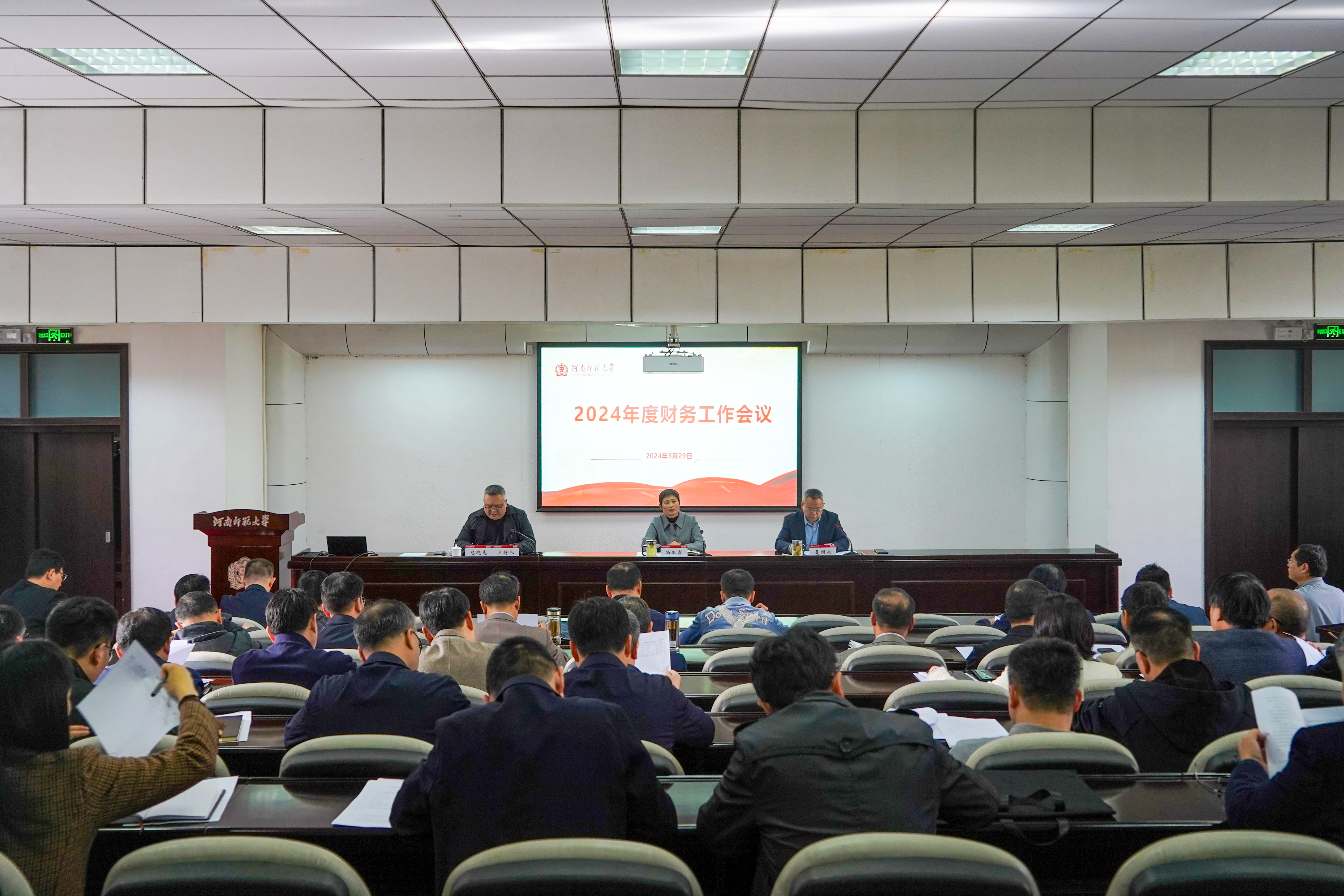