18lk新利|18新利娱乐国际
Toggle navigation about(current) blog publications opportunities Visual Interface and Behavior Exploration Lab Visual Interface and Behavior Exploration Lab --> The Visual Interface and Behavior Exploration (VIBE) Lab at Washington University in St. Louis uses interdisciplinary approaches to study visual interfaces, explore human behavior in decision-making processes, and advance the understanding of how people interact with visualizations. We utilize machine learning techniques to model human interactions with visualization tools and foster a symbiotic relationship between humans and machines. Topics of interest include: Visualization Literacy Medical Decision-Making Trust Perception Human-AI Collaboration Individual Differences people Dr. Alvitta Ottley Director Assistant Professor of Computer Science and Engineering Saugat Pandey Ph.D. Researcher Investigates visualization literacy and individual differences. Melanie Bancilhon Ph.D. Researcher Examines perception, cognitive biases, and decision-making. Jennifer Ha Ph.D. Researcher Focuses on adaptive systems and trust callibration. Oen McKinley Ph.D. Researcher Interested in for high-dimensional data. Shayan Monadjemi Alum Research Scientist at ORNL Specializes in machine learning and user modeling. news Oct 20, 2023 Paper co-autored with Leilani Battle titled “What Do We Mean When We Say Insight? A Formal Synthesis of Existing Theory” was accepted to TVCG and will be presented at VIS 2024 in Tampa! Oct 16, 2023 We are presenting two papers at VIS 2023 in Melbourne! May 15, 2023 Congratulations to Shayan Monadjemi who is now a Research Scientist at ORNL. We miss you already. May 10, 2023 Paper titled “Mini-VLAT: A Short and Effective Measure of Visualization Literacy” recieved the EuroVis 2023 best paper award! Mar 31, 2023 Paper titled “Mini-VLAT: A Short and Effective Measure of Visualization Literacy” was accepted to EuroVis and Computer Graphics Forum! selected publications What Do We Mean When We Say “Insight”? A Formal Synthesis of Existing Theory Leilani Battle, and Alvitta Ottley IEEE Transactions on Visualization and Computer Graphics, 2023 Abs HTML PDF Researchers have derived many theoretical models for specifying users’ insights as they interact with a visualization system. These representations are essential for understanding the insight discovery process, such as when inferring user interaction patterns that lead to insight or assessing the rigor of reported insights. However, theoretical models can be difficult to apply to existing tools and user studies, often due to discrepancies in how insight and its constituent parts are defined. This paper calls attention to the consistent structures that recur across the visualization literature and describes how they connect multiple theoretical representations of insight. We synthesize a unified formalism for insights using these structures, enabling a wider audience of researchers and developers to adopt the corresponding models. Through a series of theoretical case studies, we use our formalism to compare and contrast existing theories, revealing interesting research challenges in reasoning about a user’s domain knowledge and leveraging synergistic approaches in data mining and data management research. Do You Trust What You See? Toward A Multidimensional Measure of Trust in Visualization Saugat Pandey, Oen G McKinley, R Jordan Crouser, and 1 more author In 2023 IEEE Visualization and Visual Analytics (VIS), 2023 Abs PDF Few concepts are as ubiquitous in computational fields as trust. However, in the case of information visualization, there are several unique and complex challenges, chief among them: defining and measuring trust. In this paper, we investigate the factors that influence trust in visualizations. We draw on the literature to identify five factors likely to affect trust: credibility, clarity, reliability, familiarity, and confidence. We then conduct two studies investigating these factors’ relationship with visualization design features. In the first study, participants’ credibility, understanding, and reliability ratings depended on the visualization design and its source. In the second study, we find these factors also align with subjective trust rankings. Our findings suggest that these five factors are important considerations for the design of trustworthy visualizations. Mini-VLAT: A Short and Effective Measure of Visualization Literacy Saugat Pandey, and Alvitta Ottley Computer Graphics Forum, In Proceedings of the 25th EG Conference on Visualization (EuroVis), 2023 BEST PAPER AWARD Abs HTML PDF The visualization community regards visualization literacy as a necessary skill. Yet, despite the recent increase in research into visualization literacy by the education and visualization communities, we lack practical and time-effective instruments for the widespread measurements of people’s comprehension and interpretation of visual designs. We present Mini-VLAT, a brief but practical visualization literacy test. The Mini-VLAT is a 12-item short form of the 53-item Visualization Literacy Assessment Test (VLAT). The Mini-VLAT is reliable (coefficient omega = 0.72) and strongly correlates with the VLAT. Five visualization experts validated the Mini-VLAT items, yielding an average content validity ratio (CVR) of 0.6. We further validate Mini-VLAT by demonstrating a strong positive correlation between study participants’ Mini-VLAT scores and their aptitude for learning an unfamiliar visualization using a Parallel Coordinate Plot test. Overall, the Mini-VLAT items showed a similar pattern of validity and reliability as the 53-item VLAT. The results show that Mini-VLAT is a psychometrically sound and practical short measure of visualization literacy. Human-Computer Collaboration for Visual Analytics: an Agent-based Framework Shayan Monadjemi, Mengtian Guo, David Gotz, and 2 more authors Computer Graphics Forum, In Proceedings of the 25th EG Conference on Visualization (EuroVis), 2023 Abs PDF The visual analytics community has long aimed to understand users better and assist them in their analytic endeavors. As a result, numerous conceptual models of visual analytics aim to formalize common workflows, techniques, and goals leveraged by analysts. While many of the existing approaches are rich in detail, they each are specific to a particular aspect of the visual analytic process. Furthermore, with an ever-expanding array of novel artificial intelligence techniques and advances in visual analytic settings, existing conceptual models may not provide enough expressivity to bridge the two fields. In this work, we propose an agent-based conceptual model for the visual analytic process by drawing parallels from the artificial intelligence literature. We present three examples from the visual analytics literature as case studies and examine them in detail using our framework. Our simple yet robust framework unifies the visual analytic pipeline to enable researchers and practitioners to reason about scenarios that are becoming increasingly prominent in the field, namely mixed-initiative, guided, and collaborative analysis. Furthermore, it will allow us to characterize analysts, visual analytic settings, and guidance from the lenses of human agents, environments, and artificial agents, respectively. Why Combining Text and Visualization Could Improve Bayesian Reasoning: A Cognitive Load Perspective Melanie Bancilhon, Amanda Wright, Sunwoo Ha, and 2 more authors In Proceedings of the 2023 CHI Conference on Human Factors in Computing Systems, 2023 Abs PDF Investigations into using visualization to improve Bayesian reasoning and advance risk communication have produced mixed results, suggesting that cognitive ability might affect how users perform with different presentation formats. Our work examines the cognitive load elicited when solving Bayesian problems using icon arrays, text, and a juxtaposition of text and icon arrays. We used a three-pronged approach to capture a nuanced picture of cognitive demand and measure differences in working memory capacity, performance under divided attention using a dual-task paradigm, and subjective ratings of self-reported effort. We found that individuals with low working memory capacity made fewer errors and experienced less subjective workload when the problem contained an icon array compared to text alone, showing that visualization improves accuracy while exerting less cognitive demand. We believe these findings can considerably impact accessible risk communication, especially for individuals with low working memory capacity. A Unified Comparison of User Modeling Techniques for Predicting Data Interaction and Detecting Exploration Bias Sunwoo Ha, Shayan Monadjemi, Roman Garnett, and 1 more author IEEE Transactions on Visualization and Computer Graphics, In Proceedings of IEEE Visualization and Visual Analytics (VIS), 2022 Abs PDF The visual analytics community has proposed several user modeling algorithms to capture and analyze users’ interaction behavior in order to assist users in data exploration and insight generation. For example, some can detect exploration biases while others can predict data points the user will interact with before that interaction occurs. Researchers believe this collection of algorithms can help create more intelligent visual analytics tools. However, the community lacks a rigorous evaluation and comparison of these existing techniques. As a result, there is limited guidance on which method to use and when. Our paper seeks to fill this gap by comparing and ranking eight user modeling algorithms based on their performance on a diverse set of four user study datasets. We analyze exploration bias detection, data interaction prediction, and algorithmic complexity, among other measures. Based on our findings, we highlight open challenges and new directions for analyzing user interactions and visualization provenance. Contact Prof. Alvitta Ottley via email at [first_name]@wustl.edu. © Copyright 2024 Visual Interface and Behavior Exploration Lab .
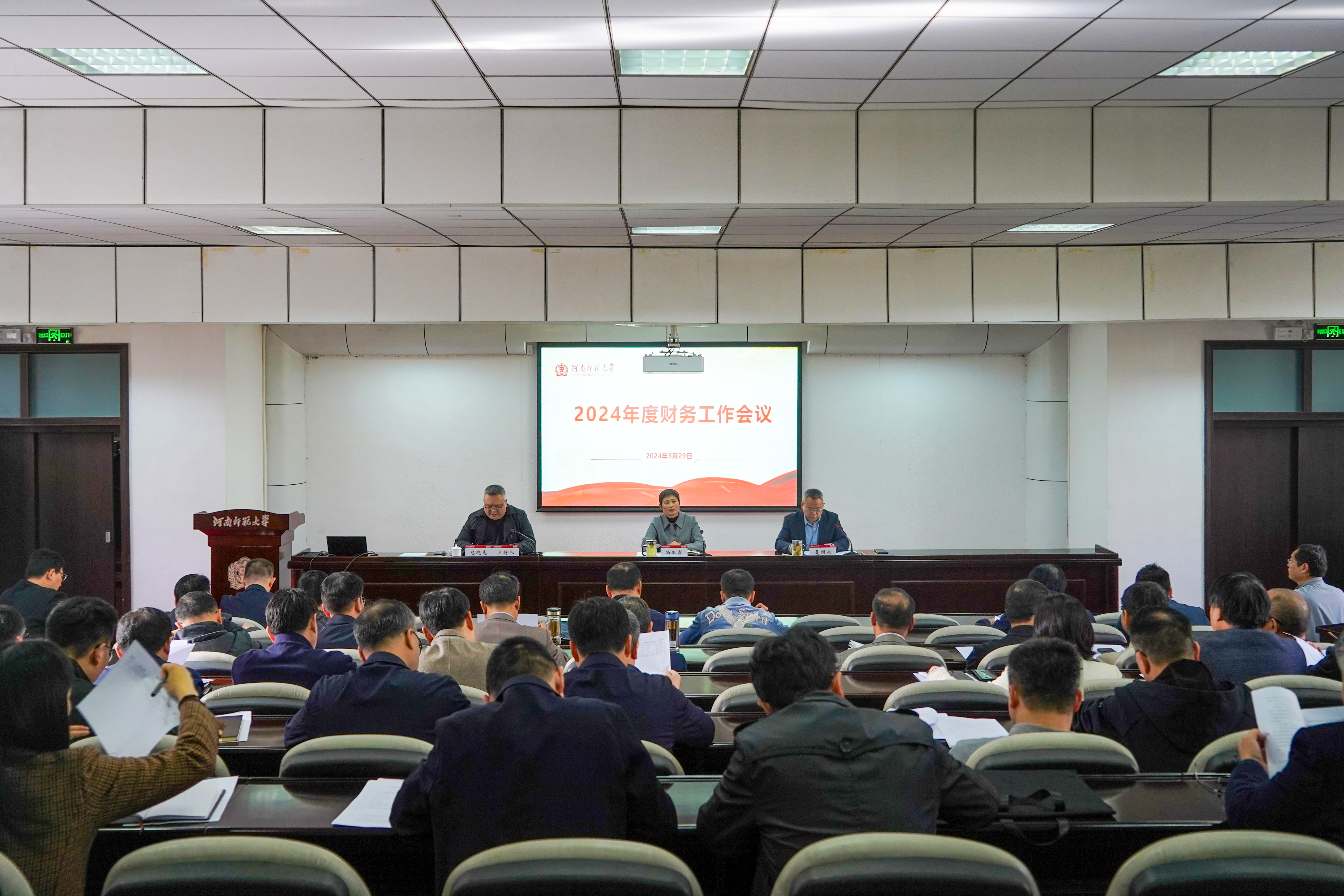